This benchmark report is set to be an exciting journey. We’ll begin by exploring key performance benchmarks and wrap up with a forecast of Nvidia’s stock price (please note: this is not financial advice).
Second Generation Benchmark - Early Preview
DeepSeek r1
Cost and Price Dynamics of DeepSeek r1
LLM Benchmark Gen2 - Early Preview
In recent months, we’ve been heavily revising our first-generation LLM Benchmark. Gen1 focused on business workload automation but relied on insights from AI use cases completed in 2023.
In the final months of the benchmark results, this reliance began to show as saturation in the top scores (with too many models achieving high marks). Additionally, the test cases had become somewhat outdated. They no longer reflected the latest insights gathered over the past year through our AI research and practical work with companies in the EU and USA.
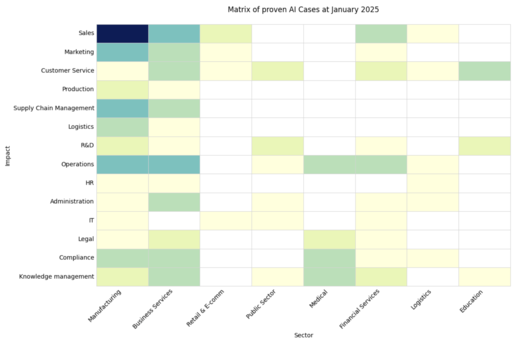
So we’ve been building a new generation of benchmark to incorporate both new LLM capabilities and new insights. The timing was just perfect—o1 pro came out to challenge the complexity of the benchmark, while DeepSeek r1 shortly thereafter introduced the concept of accessible reasoning.
Here’s an early preview of our v2 benchmark. It may not seem like much on the surface, but it already deterministically compares models on complex business tasks while allowing each model to engage in reasoning before delivering an answer.

We’ll go into the DeepSeek r1 analysis in a bit; for now, let’s focus on the benchmark itself.
Here’s the current progress and an overview of what we plan to include:
Current progress:
- ~10% of relevant AI cases are currently mapped to v2.
- As we progress towards 100%, results will become more representative of AI/LLM applications in modern business workflows.
Structured Outputs:
- Using structured outputs (following a predefined schema precisely) is a common industry standard.
- Supported by OpenAI, Google, and local inference models, making constrained decoding a key feature of our benchmark.
- Locally capable models are included wherever applicable.
Focus on business tasks:
- Benchmarks currently focus on tasks requiring multiple logical steps in a single prompt.
- Not every complex AI project needs full creative autonomy—this is counterproductive for tasks like regulatory compliance.
- Smaller, locally deployable models can often perform better in such cases by following an auditable reasoning path.
Future additions:
- We will reintroduce simpler logical tasks over time.
- A new "plan generation" category will be added, allowing for deeper analysis of workflow-oriented LLM use.
- Our ultimate goal: evaluate if powerful, cloud-based models can be replaced by simpler, local models that follow structured workflows.
Customer and partner insights:
- Customers and partners have requested access to benchmark details for inspiration and guidance in their projects.
- Unlike v1, v2 will include clean, non-restricted test cases that we can share upon request.
Current categories:
- Only a few categories of test cases are included so far.
- Additional categories will be added as the benchmark evolves.
The full journey will take a few months, but the hardest part—bringing many moving pieces together into a single coherent framework—is done!
From here on, LLM Benchmark v2 will only continue to improve.
DeepSeek r1
Let’s talk about the elephant in the room. DeepSeek r1 is the new Chinese model, much faster and cheaper than OpenAI’s winning o1 model. In addition to being locally deployable (anyone can download it), it is also designed to be smarter.
No surprise that stocks took a hit after these developments.
Let’s start with its reasoning capabilities. According to our benchmarks, DeepSeek r1 performs exceptionally well:
- It outperforms almost all variants of OpenAI’s 4o models.
- It surpasses any open-source model.
- However, it still lags behind OpenAI’s o1 and GPT-4o (August 2024 edition).
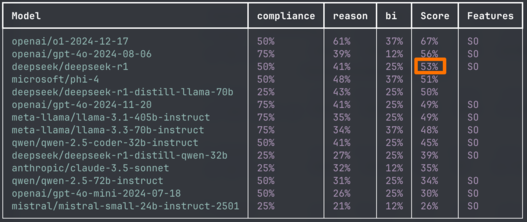
Also remember that the base DeepSeek r1 is a Mixture of Experts model containing 685B parameters in total (which means you’ll need sufficient GPU capacity to handle them all). When comparing its score progression to other large open-source models, the progress appears roughly proportional to its size.
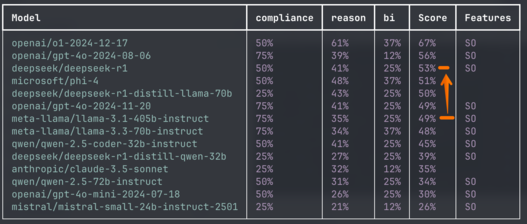
Do you see a smaller elephant in the room that breaks this pattern? It’s the distillation of DeepSeek r1’s capabilities into Llama 70B! This locally deployable model isn’t the one everyone is focusing on, but it could potentially be the most significant development.
If you can enhance any good foundational model by distilling r1’s reasoning capabilities and allowing it to reason before producing an answer, it presents an attractive alternative. This approach could make common models faster and more efficient.
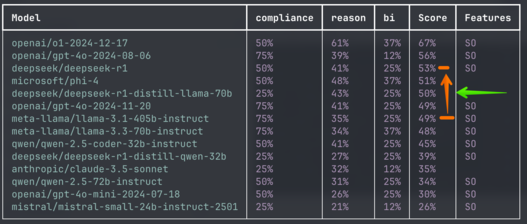
To summarise:
The DeepSeek r1 model is really good, but it’s not yet good enough to directly compete with OpenAI’s o1. Its immediate challenge is to outperform OpenAI’s 4o models before moving on to higher competition.
The technology behind DeepSeek r1 is promising and will likely lead to a new generation of more efficient reasoning models derived from distillation approaches built on its foundation. This aligns with a prediction we made in December: AI vendors will increasingly provide reasoning capabilities similar to OpenAI’s o1 models as a shortcut to quickly improve model performance. The idea is simple—allocate more compute, let the model take longer to reason before answering, and charge more for API usage. This workaround allows for accuracy improvements without requiring heavy investments in training new foundational models.
However, we also foresee that the current hype around smart reasoning models that are extremely expensive will eventually fade. Their practicality is limited, and they will likely give way to more cost-effective solutions.
Cost and Price Dynamics of DeepSeek r1
DeepSeek r1 offers a cost-effective pricing model. The cost per 1 million input tokens is just $0.55, while 1 million output tokens are priced at $2.19. This affordability positions it as a competitive option in the market, especially for those seeking locally deployable AI models.
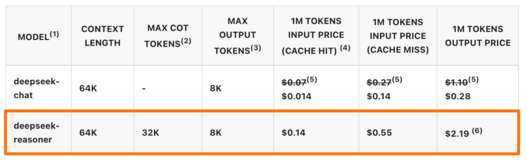
This is significantly cheaper than OpenAI’s o1 or 4o pricing. Let’s break it down in a table to make things clearer.
We’ll also calculate the total price for a common business workload with a typical 10:1 ratio—10 million input tokens and 1 million output tokens. This ratio is common in data extraction and retrieval-augmented generation (RAG) systems, which are prevalent in our AI use cases.
Model | 1M Input Tokens | 1M Output Tokens | Cost of 10M:1M |
---|---|---|---|
DeepSeek r1 | $0.55 | $2.19 | $7.69 |
OpenAI gpt-4o | $2.5 | $10 | $35 |
OpenAI o1 | $15.0 | $60 | $210 |
At this point, we can confidently say that the pricing of DeepSeek r1 blows everyone else out of the water. It’s not just “25x cheaper than OpenAI o1” for common business workloads—it’s 27x cheaper.
However, the devil is in the details. The currently offered price might not perfectly reflect the actual market price or the real cost of running the business due to various factors.
First of all, can DeepSeek even handle all the demand? According to their status page, the API has been in “Major Outage” mode since January 27th. This means they aren’t actually serving all LLM requests at the advertised price.
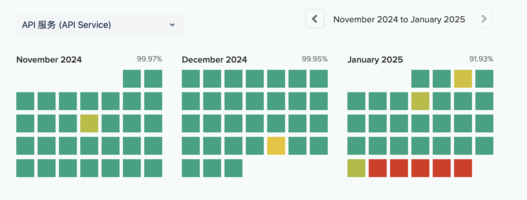
In general, if you examine the financial incentives of DeepSeek as a company, you might find that turning a profit may not be their primary motivation. DeepSeek is owned by a Chinese high-flyer hedge fund (see Wikipedia), so theoretically, they could potentially make more money by shorting Nvidia. But let’s set that theory aside for now.
Still, it’s an interesting coincidence that January 27th, the day their API entered “Major Outage” mode, is also the same day Nvidia’s stock took a nosedive.
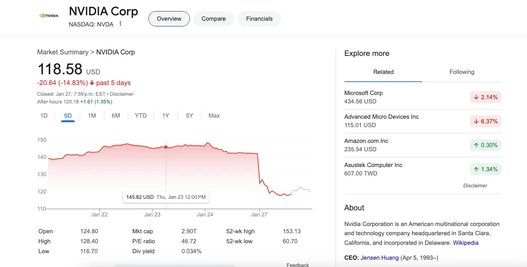
To take a deeper dive into LLM price dynamics, we can refer to a popular LLM marketplace called OpenRouter.
OpenRouter conveniently aggregates multiple providers under a single API, creating an open market for LLM-as-a-service offerings. Since DeepSeek r1 is an open-source model, multiple providers can deploy and serve it at their own pricing, allowing supply and demand to naturally balance the market.
Here’s what the pricing looks like for the top-rated providers of DeepSeek r1 at the time of writing this article (“nitro” refers to providers that can handle certain workloads):
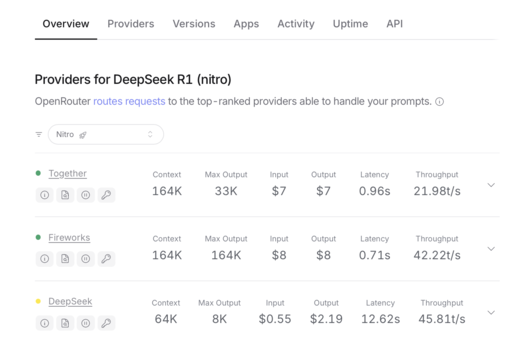
As you can see, DeepSeek attempts to offer its API at the advertised prices, but with a few nuances:
As a cost-cutting measure, it limits its input and output capacities to a fraction of what other providers offer. Compare the “Context” and “Max Output” sizes, but also keep in mind that DeepSeek’s original pricing includes a 32K Reasoning token limit in addition to an 8K Output Limit.
Normally, OpenRouter routes requests to the cheapest provider, letting market dynamics determine the flow. However, the DeepSeek r1 API hasn’t been able to keep up with the current demand and has been explicitly de-ranked with the message: “Users have reported degraded quality. Temporarily deranked.”
Meanwhile, alternative competitors—who are more motivated to generate profit—charge noticeably higher prices per input and output tokens. Despite the higher costs, they can handle the demand and maintain consistently high throughput.
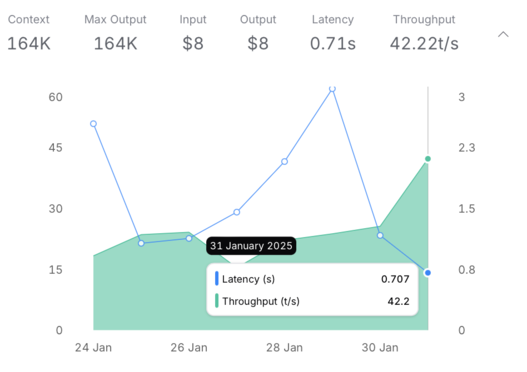
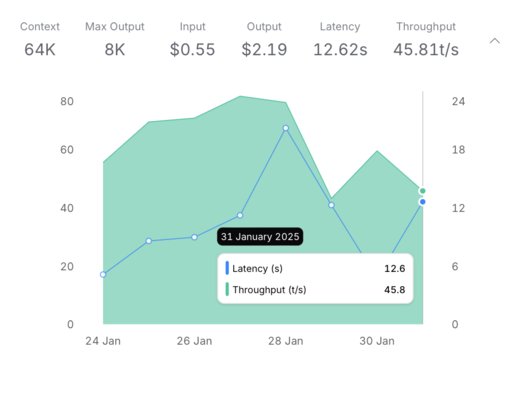
Effectively, the current market price for stable access to DeepSeek r1 is around $7 to $8 per 1M Input/Output tokens. For an average 10:1 workload (10M input tokens and 1M output tokens), this results in a total cost of $77.
This is twice as expensive as the cost of using a similarly capable GPT-4o, which comes in at $35 for the same workload.
These estimates are based on the current market price and don’t necessarily reflect the real cost of running DeepSeek r1 if you were to deploy it independently. Let’s explore the latest report from Nvidia, which highlights running DeepSeek r1 on the latest NVIDIA HGX H200 at a speed of 3,872 tokens per second using native FP8 inference to achieve this performance.
Assuming a 2-year rental cost of $16 per hour for an HGX H200 in Silicon Valley, and running the optimized software stack at ideal capacity, this results in a cost of $1.15 per 1M input/output tokens. For a 10:1 workload, that’s $12.65 per workload, which is higher than the $7.69 price that DeepSeek r1 currently advertises.
However, DeepSeek doesn’t seem to have access to the latest Nvidia hardware like the HGX H200. They are reportedly limited to H800 GPUs, an export version of the H100 with reduced memory bandwidth, which could drive their actual running costs even higher.
No matter how we analyze the numbers, the same picture emerges:
We don’t see how DeepSeek r1 could truly be 25x cheaper than OpenAI’s o1 unless its pricing is heavily subsidized. However, subsidized prices and high market demand don’t usually mix well in the long term.
On our early v2 LLM benchmark, DeepSeek r1 demonstrates reasoning capabilities comparable to an older OpenAI GPT-4o from August 2024. It’s not on the same level as OpenAI’s o1 yet.
Additionally, both OpenAI’s o1 and 4o models are multi-modal and natively support working with images and complex documents, whereas DeepSeek r1 is limited to text-only inputs. This further separates them, especially when dealing with document-oriented business workloads.
Given this, the recent stock market reaction to a promising but limited Chinese text-only model—comparable to an older OpenAI version and sold at a subsidized price—might be an overreaction. The trend toward multi-modal foundational models that understand the world beyond text is where future value lies, offering Nvidia and its partners new opportunities to generate significant returns.
Stock Market Prediction (not financial advice): Nvidia will rebound and continue to grow quickly, driven by real-world workloads and sustainable cost dynamics.
Meanwhile, the DeepSeek r1 model is still interesting and could help OpenAI’s competitors close the gap (especially since there haven’t been major innovations recently from Anthropic or Sonnet). However, DeepSeek r1 itself may fade away due to its cost dynamics, as its distilled versions are already showing stronger potential in our new Benchmark v2.
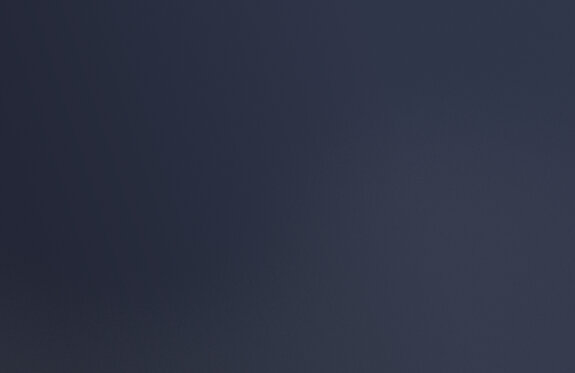
Do you want to put your RAG to the test? We are planning to run the second round of our Enterprise RAG Challenge at the end of February!
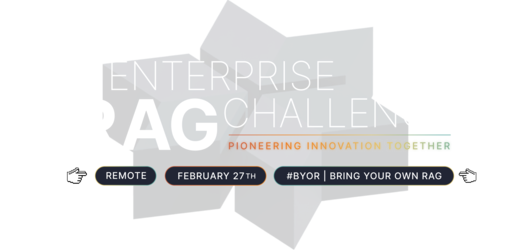
Enterprise RAG Challenge is a friendly competition where we compare how different RAG architectures answer questions about business documents.
We had the first round of this challenge last summer. Results were impressive - just with 16 participating teams we were able to compare different RAG architectures and discover the power of using structured outputs in business tasks.
The second round is scheduled for February 27th. Mark your calendars!
Transform Your Digital Projects with the Best AI Language Models!
Discover the transformative power of the best LLMs and revolutionize your digital products with AI! Stay future-focused, boost efficiency, and gain a clear competitive edge. We help you elevate your business value to the next level.
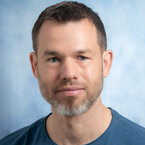
Martin Warnung
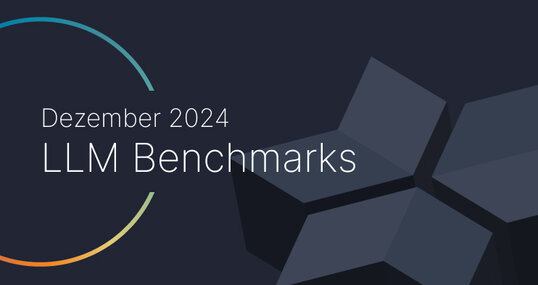
ChatGPT & Co: LLM Benchmarks for December
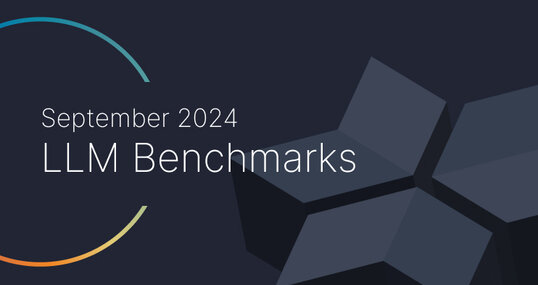
ChatGPT & Co: LLM Benchmarks for September
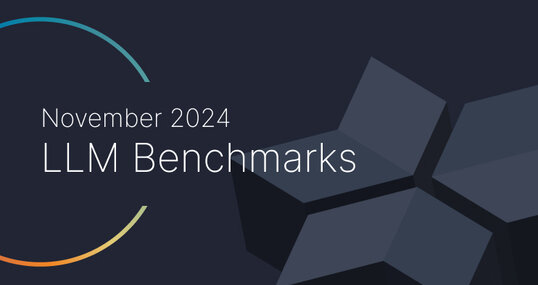
ChatGPT & Co: LLM Benchmarks for November
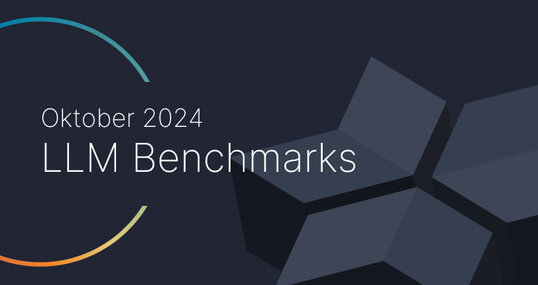
ChatGPT & Co: LLM Benchmarks for October
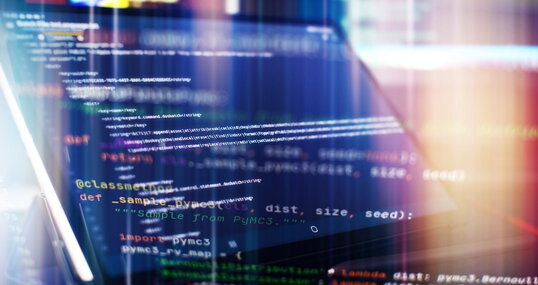
Open-sourcing 4 solutions from the Enterprise RAG Challenge
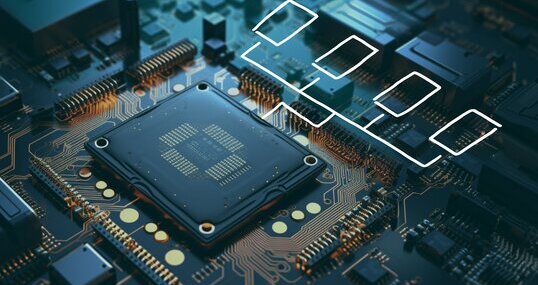
LLM Performance Series: Batching
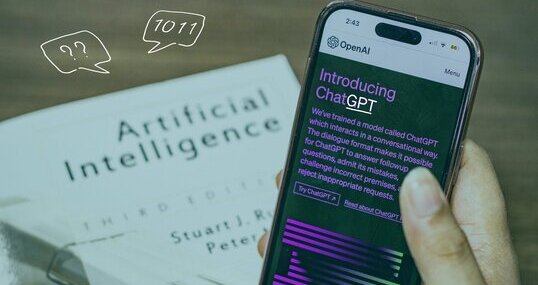
Strategic Impact of Large Language Models
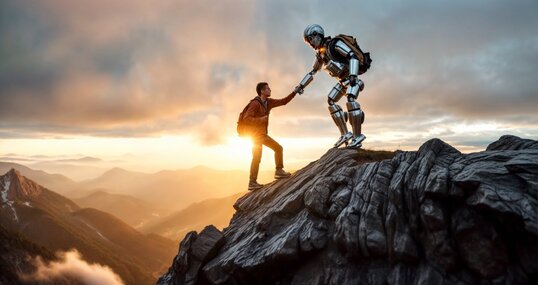
8 tips for developing AI assistants
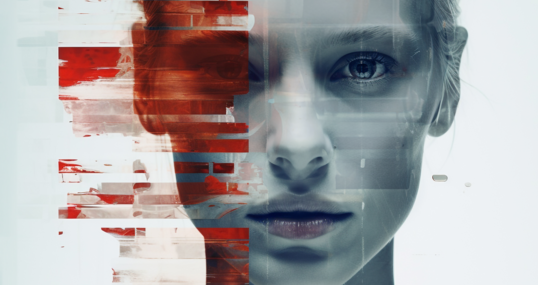
Common Mistakes in the Development of AI Assistants
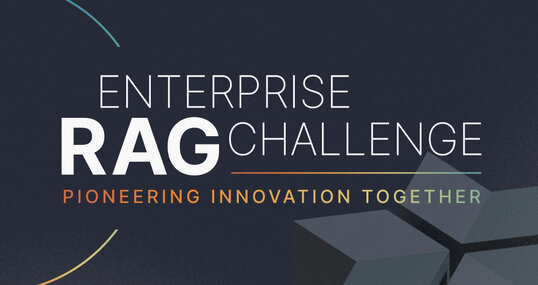
AI Contest - Enterprise RAG Challenge
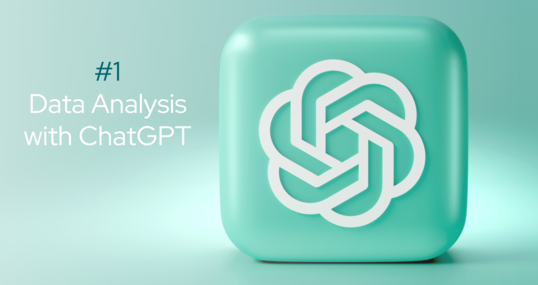
Part 1: Data Analysis with ChatGPT
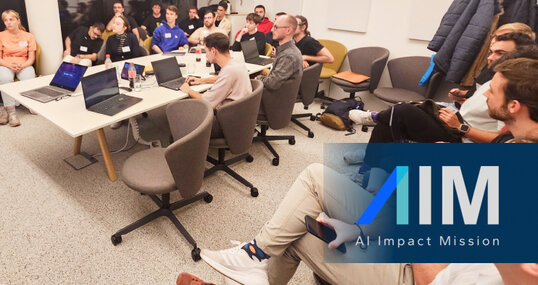
AIM Hackathon 2024: Sustainability Meets LLMs
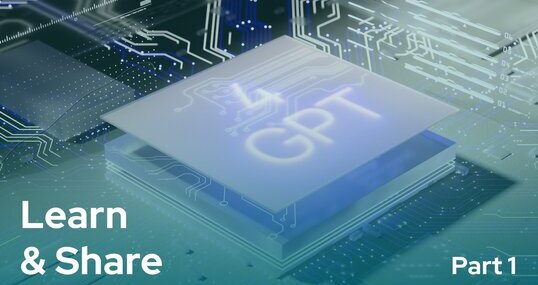
Creating a Social Media Posts Generator Website with ChatGPT
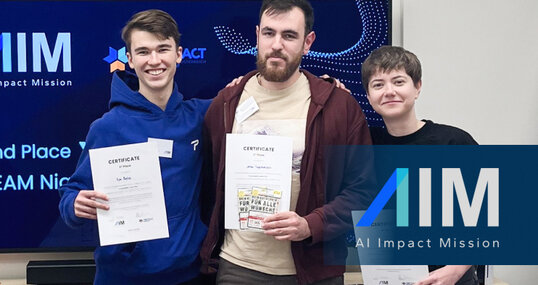
Second Place - AIM Hackathon 2024: Trustpilot for ESG
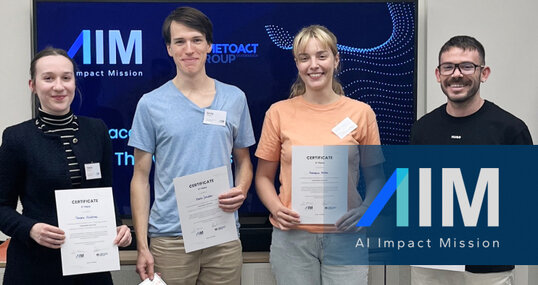
Third Place - AIM Hackathon 2024: The Venturers
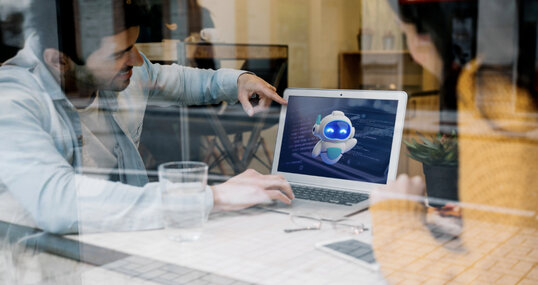
Let's build an Enterprise AI Assistant
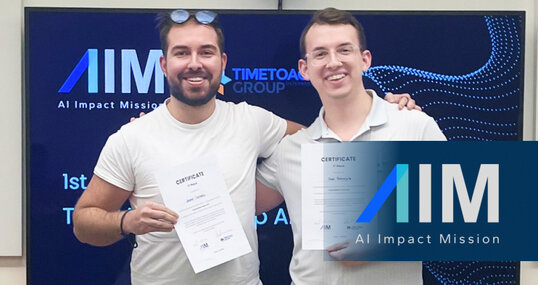
SAM Wins First Prize at AIM Hackathon
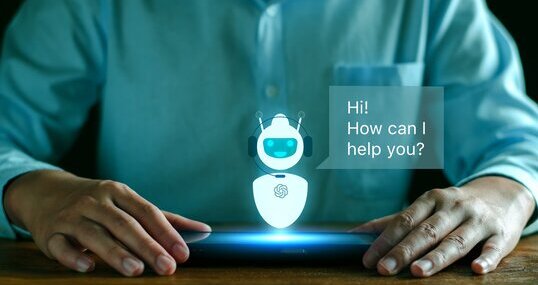
So You are Building an AI Assistant?
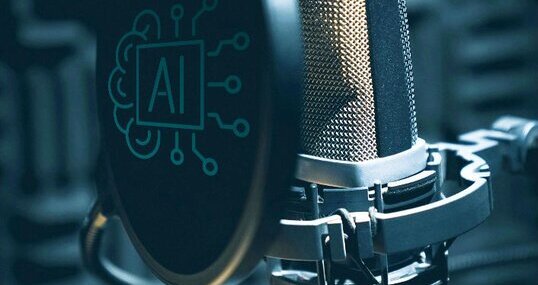
The Intersection of AI and Voice Manipulation
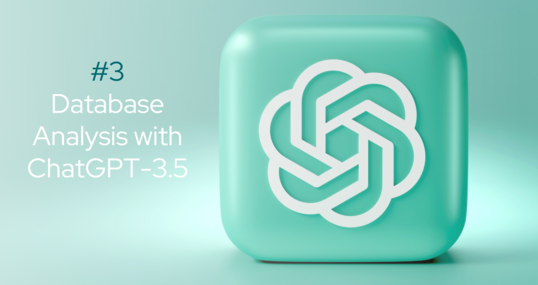
Part 3: How to Analyze a Database File with GPT-3.5
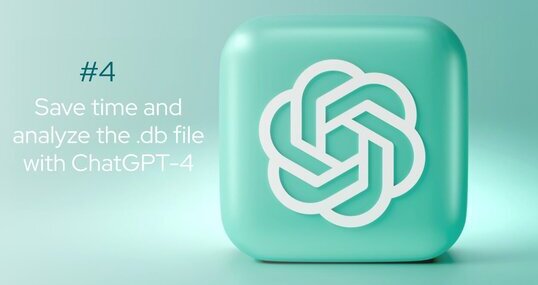
Part 4: Save Time and Analyze the Database File
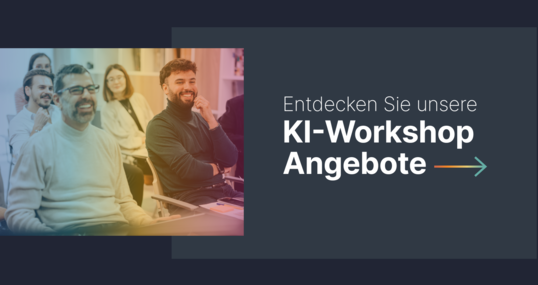
AI Workshops for Companies
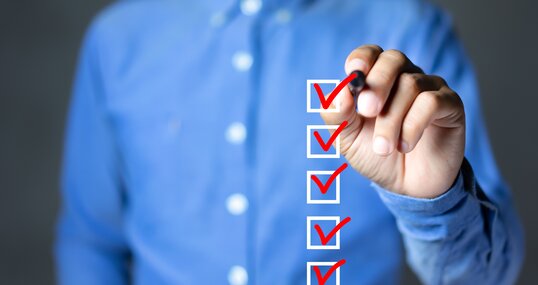
License Management – Everything you need to know
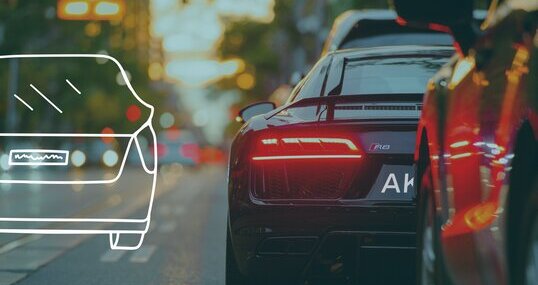
License Plate Detection for Precise Car Distance Estimation

5 Inconvenient Questions when hiring an AI company
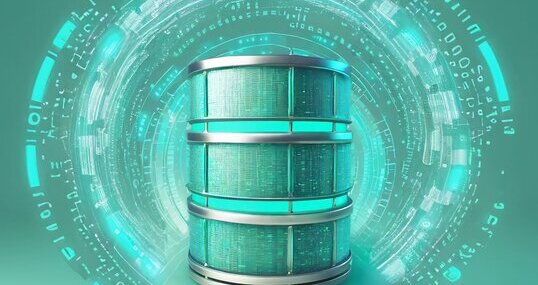
Database Analysis Report
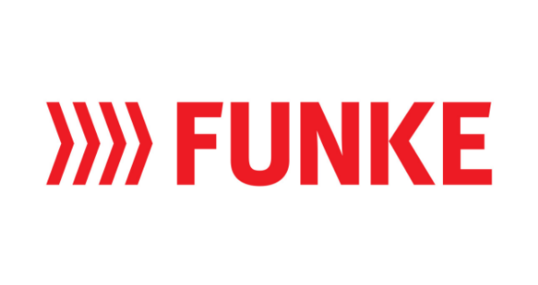
Managed service support for optimal license management
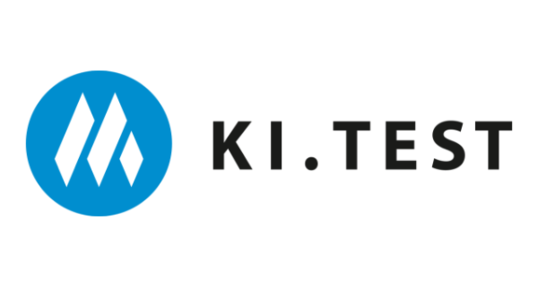
Interactive online portal identifies suitable employees
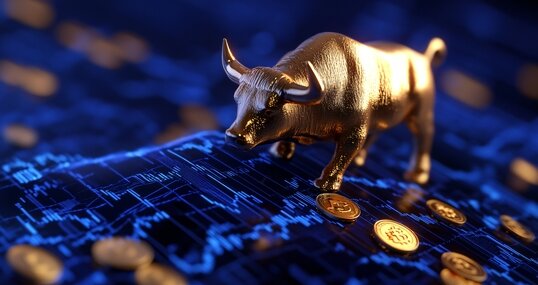
Artificial Intelligence in Treasury Management
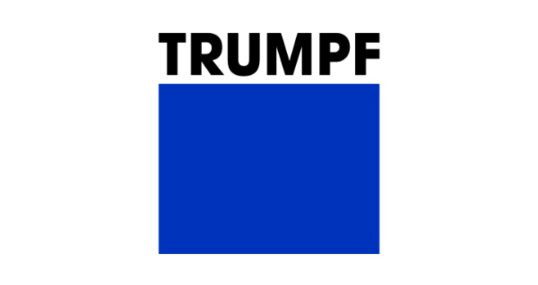
Standardized data management creates basis for reporting
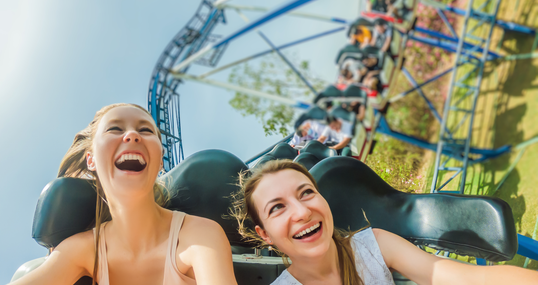
Flexibility in the data evaluation of a theme park
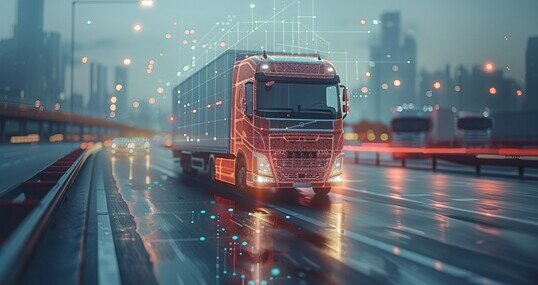
Automated Planning of Transport Routes
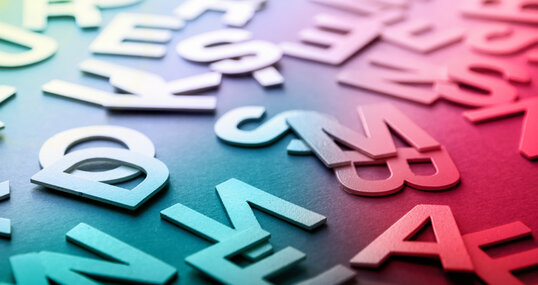
Using NLP libraries for post-processing
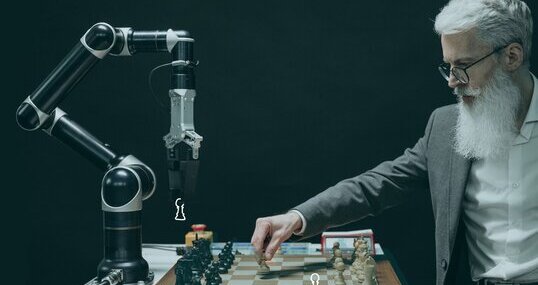
Machine Learning Pipelines
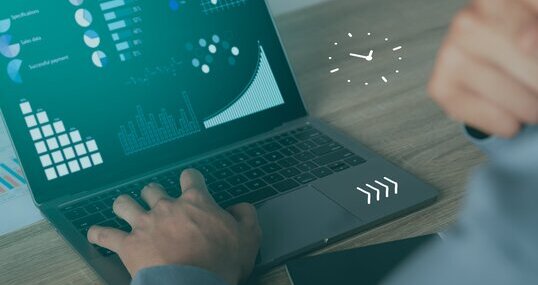
Boosting speed of scikit-learn regression algorithms

Revolutionizing the Logistics Industry
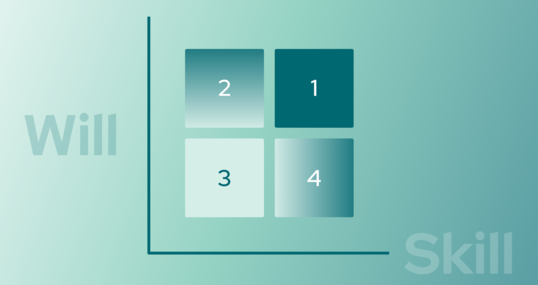
Using a Skill/Will matrix for personal career development
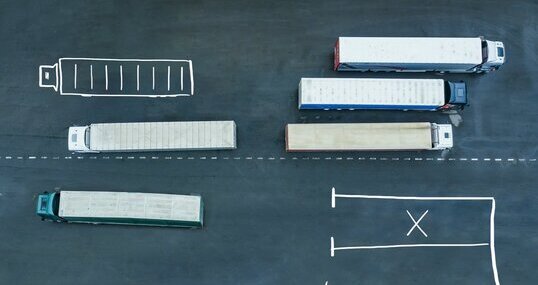
Part 2: Detecting Truck Parking Lots on Satellite Images
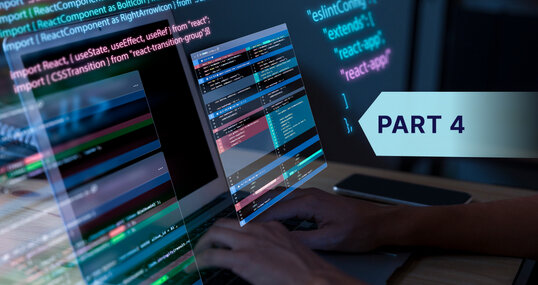
Building A Shell Application for Micro Frontends | Part 4
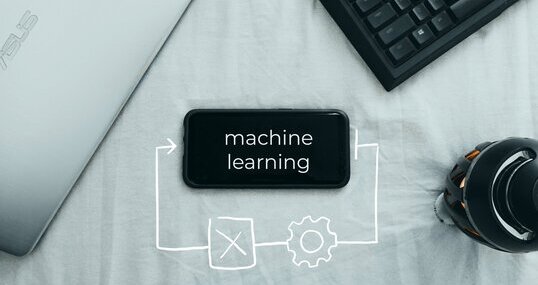
Creating a Cross-Domain Capable ML Pipeline
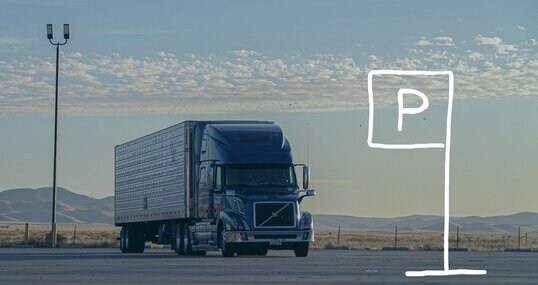
Part 1: Detecting Truck Parking Lots on Satellite Images

Introduction to Functional Programming in F# – Part 5
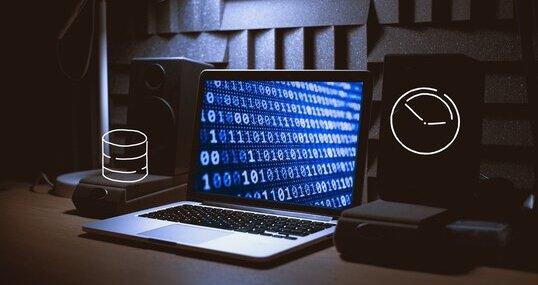
State of Fast Feedback in Data Science Projects
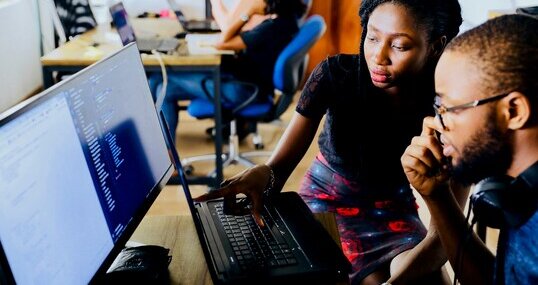
Innovation Incubator Round 1
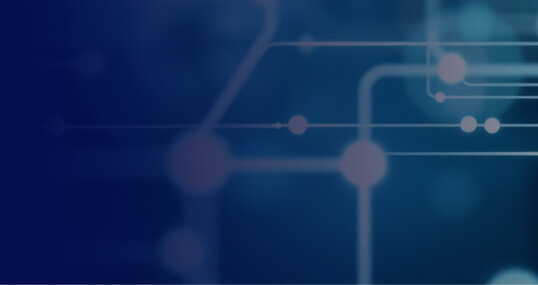
How we discover and organise domains in an existing product
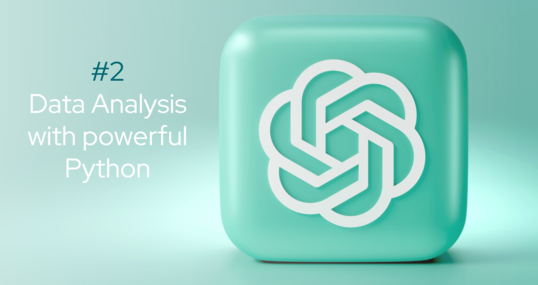
Part 2: Data Analysis with powerful Python
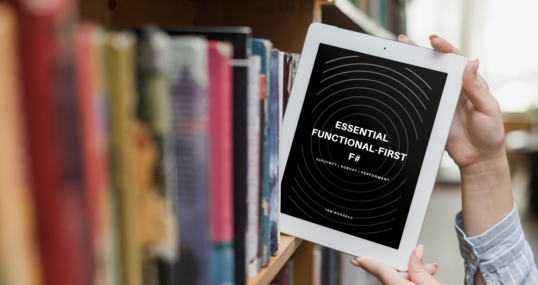
So, I wrote a book

Introduction to Functional Programming in F# – Part 8
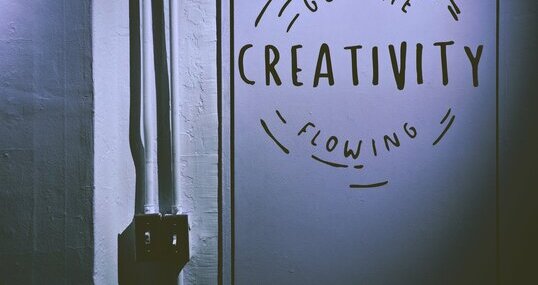
Innovation Incubator at TIMETOACT GROUP Austria
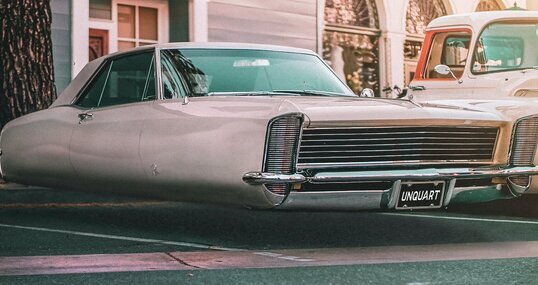
They promised it would be the next big thing!
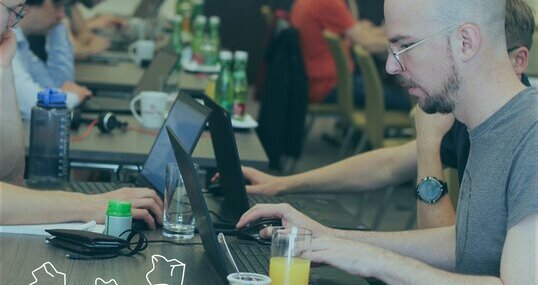
Part 1: TIMETOACT Logistics Hackathon - Behind the Scenes
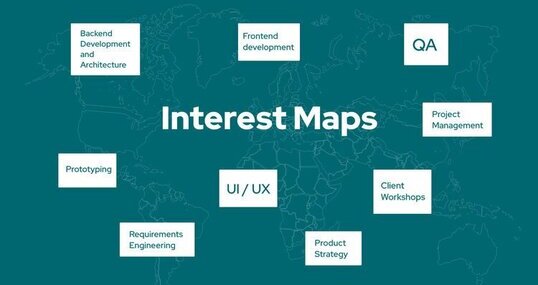
7 Positive effects of visualizing the interests of your team
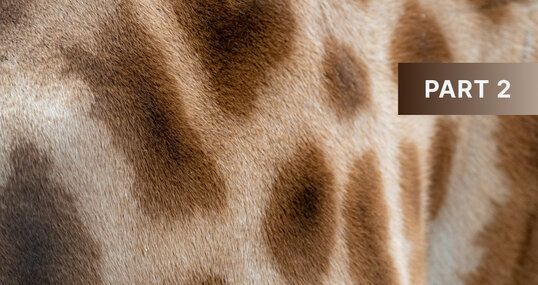
Introduction to Web Programming in F# with Giraffe – Part 2
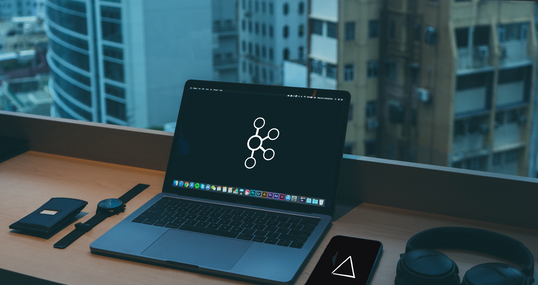
Event Sourcing with Apache Kafka
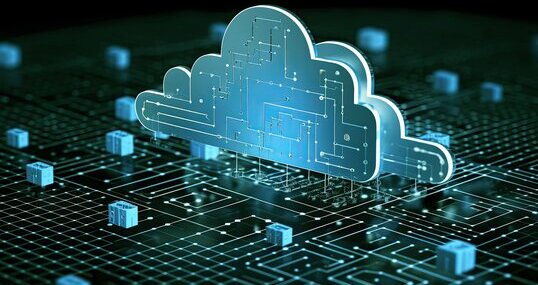
Make Your Value Stream Visible Through Structured Logging
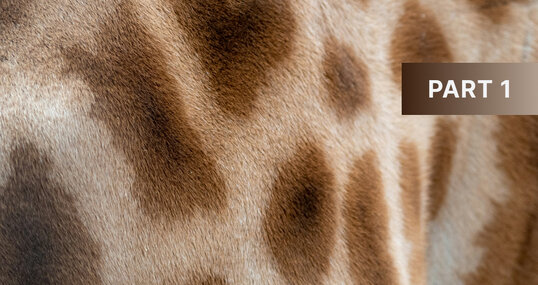
Introduction to Web Programming in F# with Giraffe – Part 1

Introduction to Functional Programming in F# – Part 6
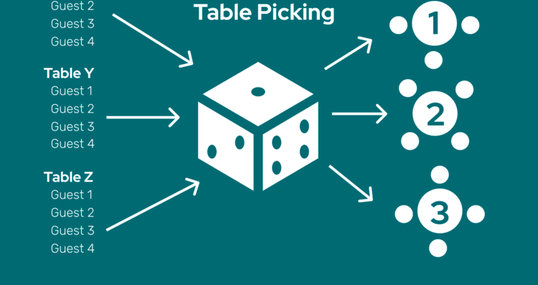
The Power of Event Sourcing

Designing and Running a Workshop series: An outline
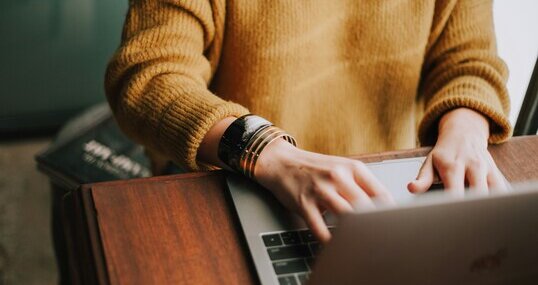
My Weekly Shutdown Routine

Introduction to Functional Programming in F# – Part 9

Introduction to Functional Programming in F#
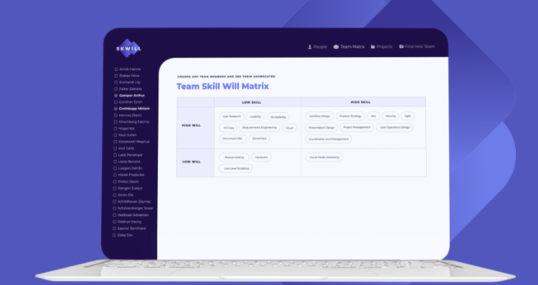
From the idea to the product: The genesis of Skwill
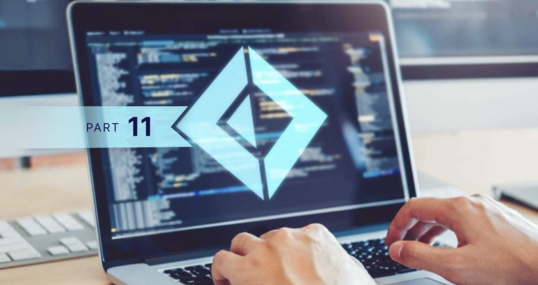
Introduction to Functional Programming in F# – Part 11
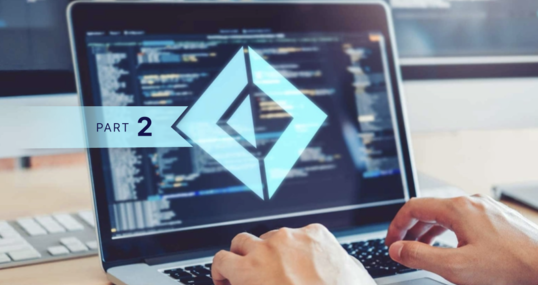
Introduction to Functional Programming in F# – Part 2
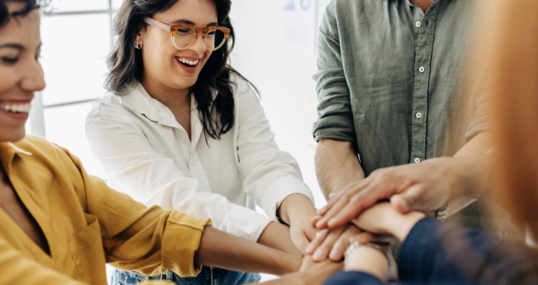
Why Was Our Project Successful: Coincidence or Blueprint?

Introduction to Functional Programming in F# – Part 10
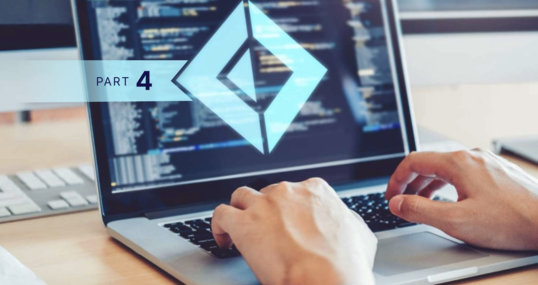
Introduction to Functional Programming in F# – Part 4
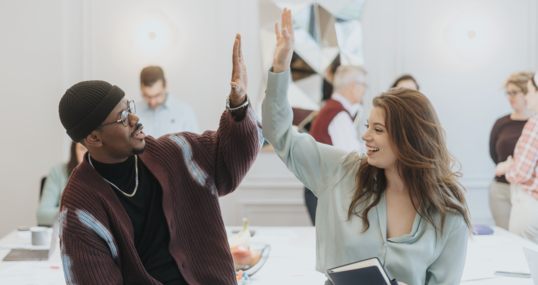
ADRs as a Tool to Build Empowered Teams
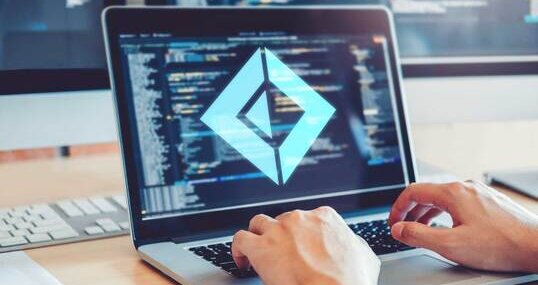
Introduction to Partial Function Application in F#

Tracing IO in .NET Core

Designing and Running a Workshop series: The board
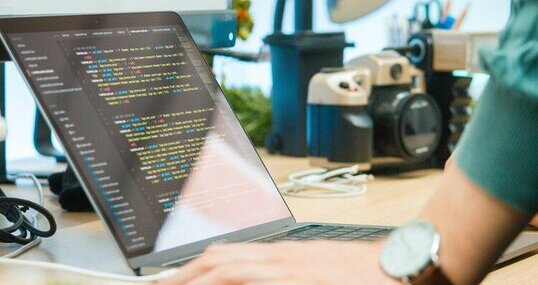
My Workflows During the Quarantine

Introduction to Functional Programming in F# – Part 7
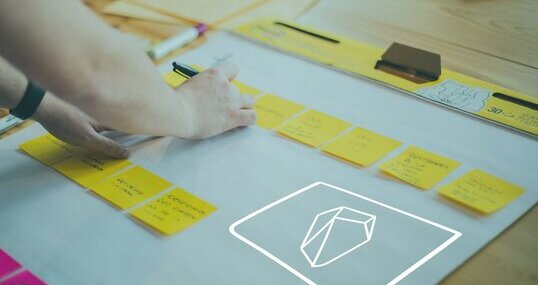
Learn & Share video Obsidian

Introduction to Functional Programming in F# – Part 3
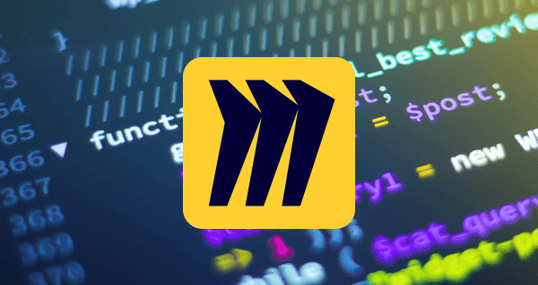
How to gather data from Miro

Introduction to Functional Programming in F# – Part 12
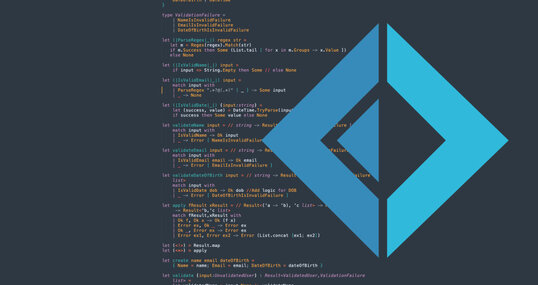
Understanding F# applicatives and custom operators
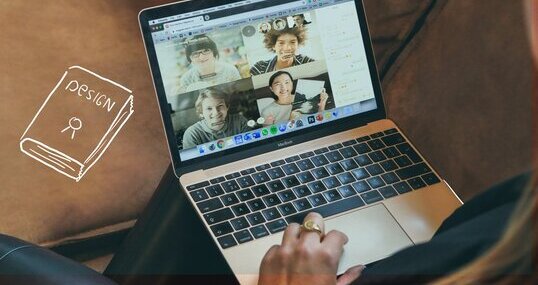
5 lessons from running a (remote) design systems book club

Running Hybrid Workshops
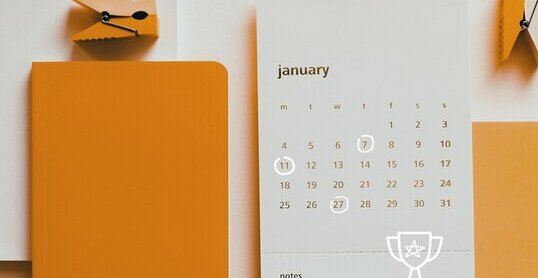